Craig Enders
cenders
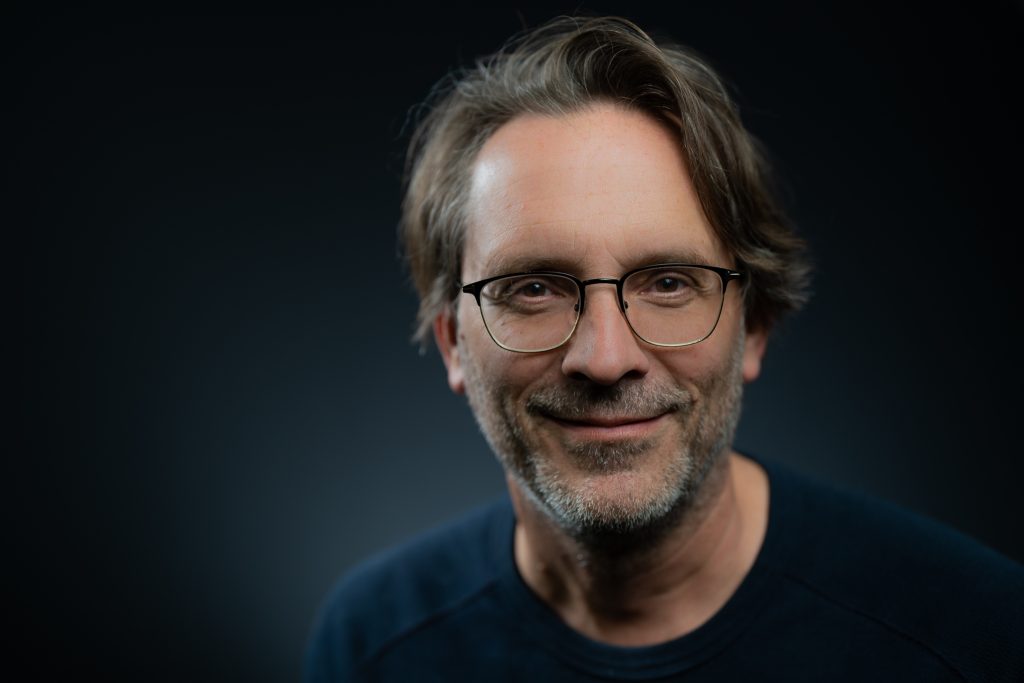
Professor
Ph.D.: University of Nebraska
Area Chair: Quantitative
Primary Area: Quantitative
Email: cenders@psych.ucla.edu
Research and Teaching Interests:
Multiple imputation, Bayesian estimation with incomplete data, multilevel modeling, longitudinal modeling.
Biography:
For the past seven years, I’ve been working on multiple imputation and Bayesian estimation approaches to missing data, with an emphasis on multilevel data structures. With support from Institute of Educational Sciences awards R305D150056 (Craig Enders, PI; Roy Levy, Co-PI) and R305D190002 (Craig Enders, PI; Brian Keller and Han Du, Co-PIs), our research team has developed the standalone software package, Blimp. Blimp was originally designed as a multiple imputation program, but the application now offers general-purpose Bayesian estimation for a wide range of single-level and multilevel regression models with two or three levels, with or without missing data. In particular, the application offers estimation routines for interactive and polynomial effects that are not yet available in other software packages, including support for binary, ordinal, multicategoricual nominal, skewed continuous variables, and count variables. The third iteration of the software extends Blimp’s capabilities to accommodate multiple equations, including structural equation and latent variable models. Blimp is freely available for macOS, Windows, and Linux at www.appliedmissingdata.com/blimp.
Curriculum Vitae
Representative Publications:
A few representative publications are shown below, and a list of Blimp-oriented papers is available at www.appliedmissingdata.com/blimp-papers.
Enders, C.K. (2022). Applied missing data analysis (2nd ed.). New York: Guilford.
Enders, C.K. (2022). Fitting structural equation models with missing data. In R. Hoyle (Ed.), Handbook of structural equation modeling (2nd ed., pp. 223-241) Guilford Press: New York.
Enders, C.K., & Hayes, T. (2022). Missing data handling for multilevel data. In A. O’Connell, B. McCoach, & B. Bell (Eds.), Multilevel modeling methods with introductory and advanced applications (pp. 535-566). Information Age: Greenwich, CT.
Keller, B. T., & Enders, C. K. (2021). Blimp user’s guide (Version 3). Available at www.appliedmissingdata.com/blimp.
Enders, C.K., Du, H., & Keller, B.T. (2020). A model-based imputation procedure for multilevel regression models with random coefficients, interaction effects, and non-linear terms. Psychological Methods, 25, 88-112.
Mansolf, M., Jorgensen, T.D., & Enders, C.K. (2020). A multiple imputation score test for model modification in structural equation models. Psychological Methods, 25, 393-411.
Enders, C.K., Hayes, T., & Du, H. (2018). A comparison of multilevel imputation schemes for random coefficient models: Fully conditional specification and joint model imputation with random covariance matrices. Multivariate Behavioral Research, 53, 695-713.
Enders, C.K., Keller, B.T., & Levy, R. (2018). A fully conditional specification approach to multilevel imputation of categorical and continuous variables. Psychological Methods, 23, 298-317.
Enders, C.K., & Mansolf, M. (2018). Assessing fit of structural equation models with multiply imputed data. Psychological Methods, 23, 76-93.
Enders, C.K. (2017). Multiple imputation as a flexible tool for missing data handling in clinical research. Behaviour Research and Therapy, 98, 4-18.
Enders, C.K., Mistler, S., & Keller, B. (2016). Multilevel multiple imputation: A review and evaluation of joint modeling and chained equations imputation. Psychological Methods, 21, 222-240.
www.appliedmissingdata.com